Machine intelligence accelerates research into mapping brains

Scientists in Japan’s brain science project have used machine intelligence to improve the accuracy and reliability of a powerful brain-mapping technique, a new study reports.
Their development, published on December 18th in Scientific Reports, gives researchers more confidence in using the technique to untangle the human brain’s wiring and to better understand the changes in this wiring that accompany neurological or mental disorders such as Parkinson’s or Alzheimer’s disease.
“Working out how all the different brain regions are connected – what we call the connectome of the brain – is vital to fully understand the brain and all the complex processes it carries out,” said Professor Kenji Doya, who leads the Neural Computation Unit at the Okinawa Institute of Science and Technology Graduate University (OIST).
To identify connectomes, researchers track nerve cell fibers that extend throughout the brain. In animal experiments, scientists can inject a fluorescent tracer into multiple points in the brain and image where the nerve fibers originating from these points extend to. But this process requires analyzing hundreds of brain slices from many animals. And because it is so invasive, it cannot be used in humans, explained Prof. Doya.
However, advances in magnetic resonance imaging (MRI) have made it possible to estimate connectomes non-invasively. This technique, called diffusion MRI-based fiber tracking, uses powerful magnetic fields to track signals from water molecules as they move – or diffuse – along nerve fibers. A computer algorithm then uses these water signals to estimate the path of the nerve fibers throughout the whole brain.

But at present, the algorithms do not produce convincing results. Just like how photographs can look different depending on the camera settings chosen by a photographer, the settings – or parameters – chosen by scientists for these algorithms can generate very different connectomes.
“There are genuine concerns with the reliability of this method,” said Dr. Carlos Gutierrez, first author and postdoctoral researcher in the OIST Neural Computation Unit. “The connectomes can be dominated by false positives, meaning they show neural connections that aren’t really there.”
Furthermore, the algorithms struggle to detect nerve fibers that stretch between remote regions of the brain. Yet these long-distance connections are some of the most important for understanding how the brain functions, Dr. Gutierrez said.
In 2013, scientists launched a Japanese government-led project called Brain/MINDS (Brain Mapping by Integrated Neurotechnologies for Disease Studies) to map the brains of marmosets -- small nonhuman primates whose brains have a similar structure to human brains.
The brain/MINDS project aims to create a complete connectome of the marmoset brain by using both the non-invasive MRI imaging technique and the invasive fluorescent tracer technique.
“The data set from this project was a really unique opportunity for us to compare the results from the same brain generated by the two techniques and determine what parameters need to be set to generate the most accurate MRI-based connectome,” said Dr. Gutierrez.
In the current study, the researchers set out to fine-tune the parameters of two different widely-used algorithms so that they would reliably detect long-range fibers. They also wanted to make sure the algorithms identified as many fibers as possible while minimally pinpointing ones that were not actually present.
Instead of trying out all the different parameter combinations manually, the researchers turned to machine intelligence.
To determine the best parameters, the researchers used an evolutionary algorithm. The fiber tracking algorithm estimated the connectome from the diffusion MRI data using parameters that changed – or mutated – in each successive generation. Those parameters competed against each other and the best parameters – the ones that generated connectomes that most closely matched the neural network detected by the fluorescent tracer – advanced to the next generation.
The researchers tested the algorithms using fluorescent tracer and MRI data from ten different marmoset brains.
But choosing the best parameters wasn’t simple, even for machines, the researchers found. “Some parameters might reduce the false positive rate but make it harder to detect long-range connections. There’s conflict between the different issues we want to solve. So deciding what parameters to select each time always involves a trade-off,” said Dr. Gutierrez.
Throughout the multiple generations of this “survival-of-the-fittest” process, the algorithms running for each brain exchanged their best parameters with each other, allowing the algorithms to settle on a more similar set of parameters. At the end of the process, the researchers took the best parameters and averaged them to create one shared set.
“Combining parameters was an important step. Individual brains vary, so there will always be a unique combination of parameters that works best for one specific brain. But our aim was to come up with the best generic set of parameters that would work well for all marmoset brains,” explained Dr. Gutierrez.
The team found that the algorithm with the generic set of optimized parameters also generated a more accurate connectome in new marmoset brains that weren’t part of the original training set, compared to the default parameters used previously.

The striking difference between the images constructed by algorithms using the default and optimized parameters sends out a stark warning about MRI-based connectome research, the researchers said.
“It calls into question any research using algorithms that have not been optimized or validated,” cautioned Dr. Gutierrez.
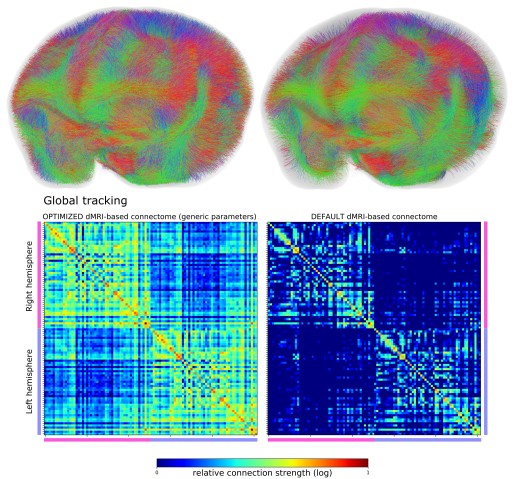
In the future, the scientists hope to make the process of using machine intelligence to identify the best parameters faster, and to use the improved algorithm to more accurately determine the connectome of brains with neurological or mental disorders.
“Ultimately, diffusion MRI-based fiber tracking could be used to map the whole human brain and pinpoint the differences between healthy and diseased brains,” said Dr. Gutierrez. “This could bring us one step closer to learning how to treat these disorders.”
###
This collaboration involved the Okinawa Institute of Science and Technology Graduate University (OIST), the RIKEN Center for Brain Science and Kyoto University. It was funded by AMED (Japan Agency for Medical Research and Development). Grant Numbers: JP18dm0207030to KD/ JP20dm0207001 to HO/ JP20dm0207088 to KN
Research Unit
For press enquiries:
Press Inquiry Form